The first Barcelona Institute for Global Health (ISGlobal) Data Science Meet-up, focused on biases in artificial intelligence, took place last Tuesday 23rd May in the Marie Curie Room, at the inner square of the Barcelona Biomedical Research Park (PRBB). These meet-ups are a series of hybrid monthly face-to-face and online talks aimed to outline different data science problems and discuss solutions. The meet-ups, organised by the Biomedical Data Science group led by Paula Petrone at ISGlobal, are open to the whole PRBB community and beyond, and also live-streamed. The meet-ups are followed by some snacks and networking time.
The monthly data science meet-ups are open to the whole PRBB community and beyond
On this occasion, the host was Pablo Iáñez Picazo, a recent La Caixa Ph.D. fellow student in the group. The guest speaker for this edition was Davide Cirillo, head of the Machine Learning for Biomedical Research Unit at the life sciences department of the Barcelona Supercomputing Center (BSC), a research centre which hosts one of the most powerful supercomputers in Europe, the MareNostrum.
Cirilo was re-visiting the PRBB years after finishing his PhD at the park, in the group led by Gian Gaetano Tartaglia, at the Centre for Genomic Regulation (CRG) back then. His current research is focused on the application of machine learning and network science for precision medicine, with a great emphasis on ethics of Artificial Intelligence (AI). In the seminar he talked about sex and gender differences and biases in AI for biomedicine and healthcare
Causes of biases in technology
Before understanding what causes bias, we need to clarify what bias is. A bias is a systematic error that results in a disadvantage for an individual or a group of people. There are innumerable biases, and, as Davide mentioned, there will always be; and new ones will even be created as new technologies are created.
Biases in technology can be related to automated processes and analytical procedures (statistical bias). But there are also biases due to the humans behind the technology: cognitive biases at the individual level, and cultural biases at societal level. All these biases can have specific attributes, for instance they can be explicit or implicit, making them much harder to detect. But how does bias appear in AI?
Data is used to train models, which can be used to make predictions that may have an impact in society. If the data used is biased, this impact will likely have negative consequences for some people. So, in the most common cases, the problem starts with the data. For this reasons, data collection is the first aspect that we should focus our attention on.
“Sadly, we live in a world where there are inequalities and discrimination. So whatever data we get out of this world will contain traces of this discrimination. And if a model is created with this data, its use will perpetuate the discrimination”
Davide Cirillo (BSC)
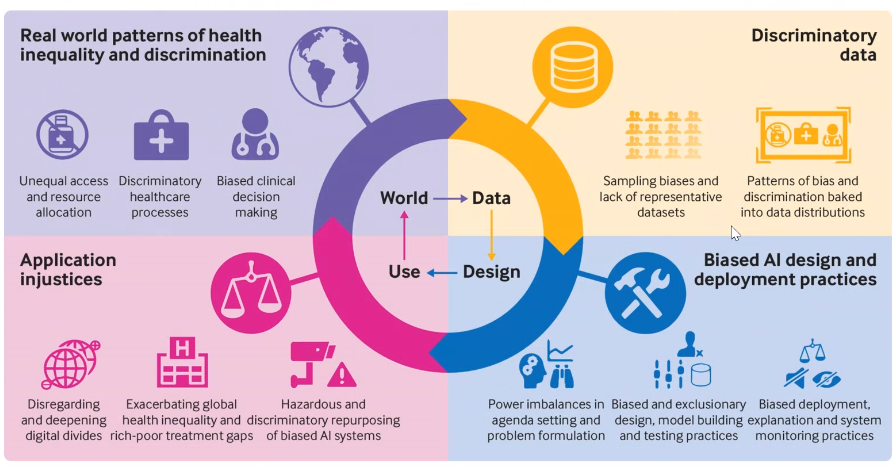
Image from Leslie et al. BMJ, vol. 372, Mar. 2021, p. N304. DOI: 10.1136/bmj.n304.
What to do about it
The data used for AI development in biomedicine may come from different places. For instance, one of the largest repositories of human data in Europe is EGA (European Genome-Phenome Archive).
Davide and a team of researchers at the ELIXIR Biohackathon Europe anlysed the metadata of EGA to see if the sex categories were properly reported in the collected samples. They also examined dbGaP, the US counterpart of EGA. The comparison of the USvand European databases showed an underreporting of biological sex of the samples collected in dbGaP. Indeed, in the US repository, almost half of the studies contained samples from unknown sex, while in the European repository almost half of the studies have samples properly reported as female or male. A notable indication of this improvement in the European version is that the EGA mandated the sex category for all data submissions in 2018.
“Data collectors and databases play a crucial role in assessing the sex/gender classification of the data in order to prevent issues later on”
In addition to the metadata analysis, Davide and his colleagues surveyed the participants of the ELIXIR Biohackathon Europe, all of them users of such biomedical data repositories, about their knowledge on diversity issues in biomedicine. Although they all agreed it was important to include diversity, most (67%) said they didn’t know where to find the guidelines to do that.
Thus, Davide and collaborators propose to extend the concept of the FAIR principles for data stewardship (that research data should be Findable, Accessible, Interoperable and Reusable) by adding an extra letter: an X indicating that data should also be unbiased.
To achieve FAIR-X data, it is essential to have a clear definition of sex and gender and consider non-binarism; to use standards; to guarantee transparency but also privacy preservation; and to foster education, social impact and the involvement of stakeholders.
Last year, Davide and colleagues participated in a document presented to the European Parliament about policy optionsto regarding technological bias in biomedical datasets. The document proposed to:
- Not create more regulations for this: the AI act is being discussed already, and the topic of bias should be included in that (and all other) regulations.
- Focus on data collection, e.g. promoting database certification to ensure that a source of data is unbiased.
- Be transparent, especially regarding the people from whom the data comes from.
Beyond sex and gender – a multiplicity of biases
As mentioned at the beginning, there are many types of biases beyond sex/gender, thus we need a diversity of data regarding origin, age, disabilities,… all of these characteristics that tend to have biases linked to them. “There are chains of biases, they are interlinked and nested. Intersectionality is very relevant. Beyond sex and gender bias, race bias is the most critical, but there are many more, too”, admits Davide.
“Biases they are interlinked and nested. Intersectionality is very relevant. Beyond sex and gender bias, race bias is the most critical, but there are many more, too”
Standards and metadata models are very important in this context, so the data can be properly attributed and used in a fair way. But even if that issue was solved, further problems remain:
- How to balance the presence of information regarding sex, gender, race, etc. with individuals’ right to withhold such information?
- Disaggregating the data by sex and other characteristics leads to smaller datasets, which reduces statistical power.
- Sex does not only depend on your chromosomes, but also your hormones. How to account for individuals such as transgender people, undergoing hormone therapy, or intersex people, when performing these analyses?
When it comes to sex and gender data analysis, Davide’s general recommendation is to make an effort in identifying potential sex and gender differences, which could be present or not in the dataset under study. “But the trick is to look actively for them, otherwise you’ll miss them. There are differences that you don’t see if you don’t look for them”, explains Davide.
Obviously, having a more diverse staff working in science and technology helps in detecting and mitigating these biases. “We may not eliminate bias, but we can be more bias-aware“, concludes the speaker of this first data science meet-up.
The next meet-up, on 21st June at 1’30pm, will focus on ChatGPT: applications, limitations, ethics, and much more. Stay tuned!
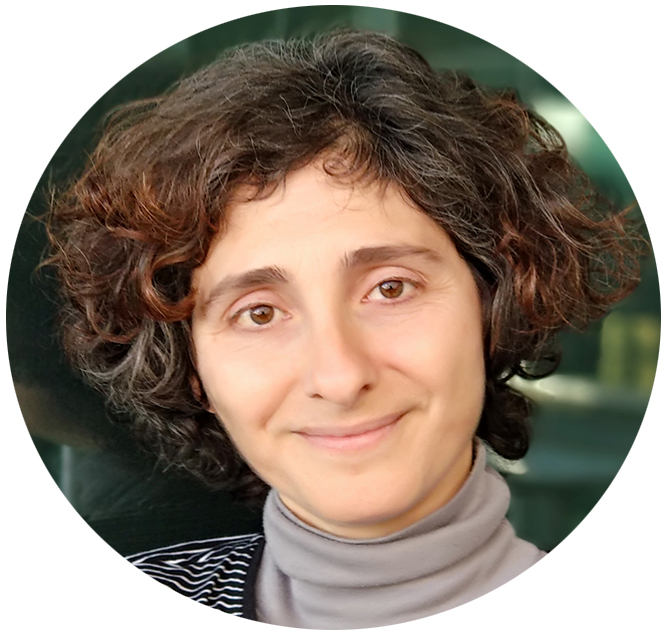