Each person has a unique DNA sequence of 3 billion nucleotides. Occasionally, one nucleotide is randomly changed for another, transmitted to the offspring and, after many generations, becomes frequent in the population. Such a mutation is known as a single nucleotide polymorphism (SNP). It has been estimated that there are about 80 million SNPs in human populations across the world. As SNPs are the most frequent form of genetic variation, researchers believe that different combinations of SNPs can help explain common heritable traits or diseases.
In recent decades, technology has made great advances, including the possibility of cheaply measuring a few million SNPs in single individuals. Bioinformaticians add algorithms to infer, from the observed SNPs, the remaining tens of millions of these polymorphisms. Research centres now bring together genome-wide SNP data from hundreds of thousands of individuals to determine which SNPs are found most frequently in which traits. Genome-wide association studies (GWASs) on diseases like cancer, mental disorders, and cardiovascular disease, as well as common traits like height or intelligence, have been performed to identify the SNPs that can help explain their heritability.
Quantitative traits, such as height or intelligence, are best explained by the small additive effects of many SNPs.
The intensive GWAS-based research has produced some important results. In general, quantitative traits are best explained by the small additive effects of many SNPs. That is, there is no “intelligence gene”, but rather a genome: the heritability of intelligence could be explained by the added contribution of all SNP variability in the genome. However, several specific findings, such as those at the FTO or APOE genes, suggest possible mechanisms of action in the development of diseases like obesity and Alzheimer’s.
The goal of GWASs is to check whether there are any SNPs, amongst millions, that can be associated to a particular disease.
The main goal of GWASs is to check whether there are any SNPs, amongst millions, that can be associated to a particular disease; it does not automatically mean that the associated SNPs (if any) are the cause of the disease. Rather, they are a first clue about where we should look further. The results are statistical in nature; they do not test biological mechanisms, and need posterior interpretation and examination. GWASs can therefore be regarded as novel empirical tools, derived from the analysis of big data, for generating informed scientific hypotheses.
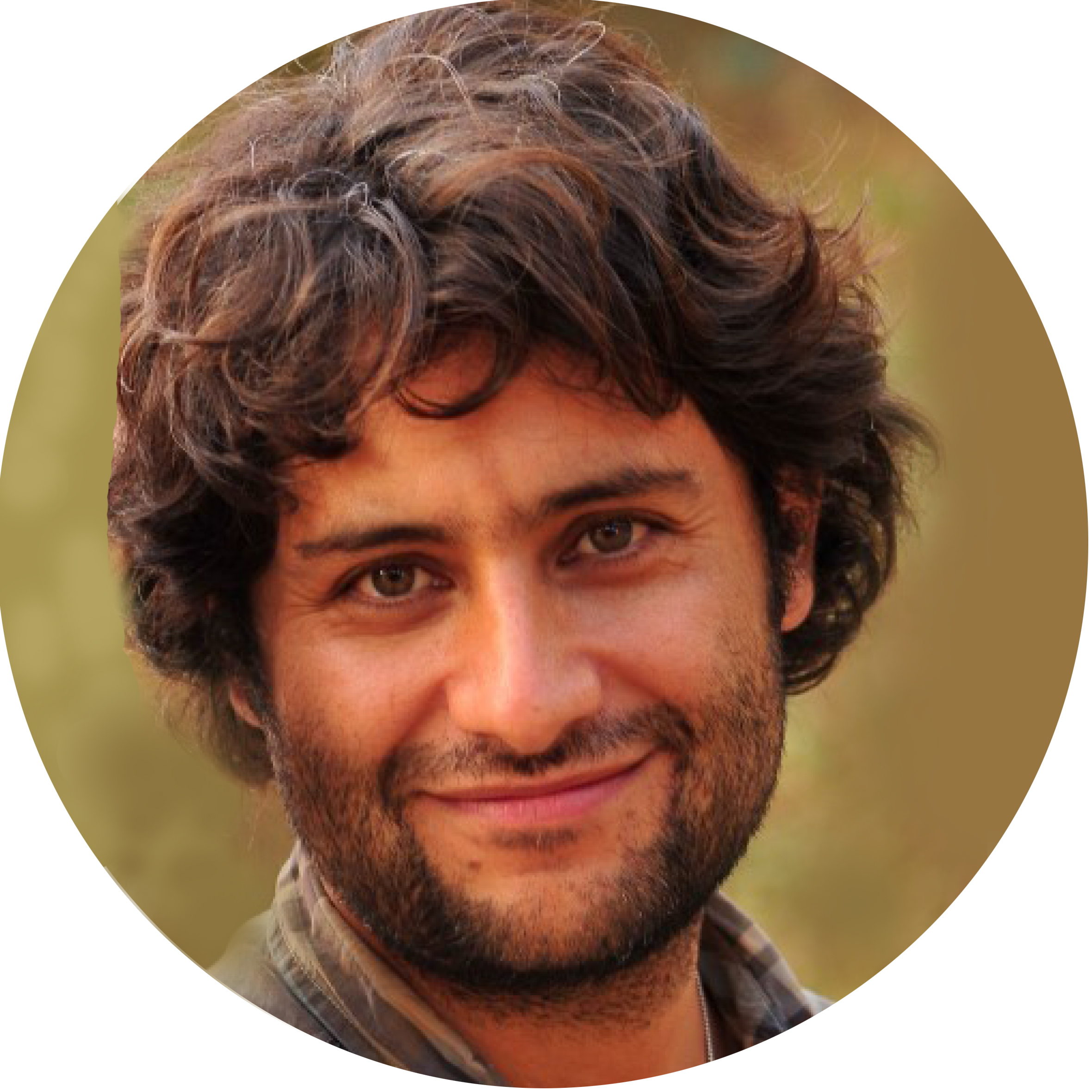